Executive Summary
Progress in treatment of upper extremity (UE) paresis after stroke has been slow. We argue that a major impediment is the lack of a direct measure of the outcomes of interest: productive use of the UE in everyday life. Current UE outcomes substitute proxies for direct measures: self-report, in-lab motor performance, and wrist-worn accelerometers measuring total movement or asymmetry of movement between the two UE’s. Thus, clinicians and clinical trialists simply do not know if their treatments work given current measures.
We have shown, in persons with stroke and UE amputation, that data from a single wrist- worn sensor analyzed with machine learning algorithms can accurately classify UE movement into functional and non-functional types when compared to ground truth determined by frame by frame analysis of video of activity performance. Moreover, our data indicate that current approaches to analyzing wrist-worn accelerometer data are inaccurate and insensitive to large differences in amounts of function determined by video ground truth. They are thus psychometrically unsuitable for use in clinical trials or treatment.
We have two Aims. First, we will increase the accuracy and usability of our data science techniques: optimize feature space, test unsupervised learning algorithms to avoid video scoring, and evaluate generalizability. Second, we will develop our approach into a clinical tool by determining its psychometric properties: test-retest reliability, convergent validity, and other properties. We will use advances in data science and sensors to accelerate progress in motor restoration by developing such a measure. We anticipate future use in other UE conditions.
Expected Deliverables
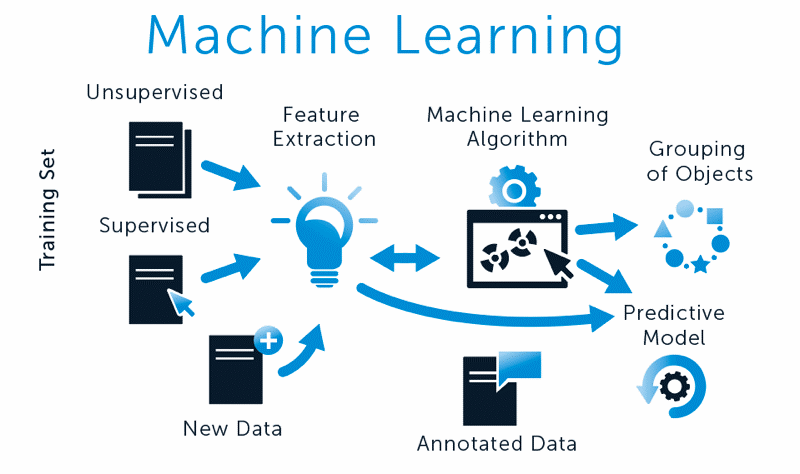
Meet the R2 Team
-
Shashwati Geed, PT, PhD
Learn More -
Lin-Ching Chang, DSc
Learn More -
Peter Lum, PhD
Learn More -
Alexander Dromerick, MD (1958-2021)
Learn More